Back to: Data Life Cycle
The research data life cycle concept must be adapted to the specific needs of a research project. For research involving bioimaging techniques, a data life cycle for orientation is proposed below (adapted from Schmidt C and Ferrando-May E, 2021).
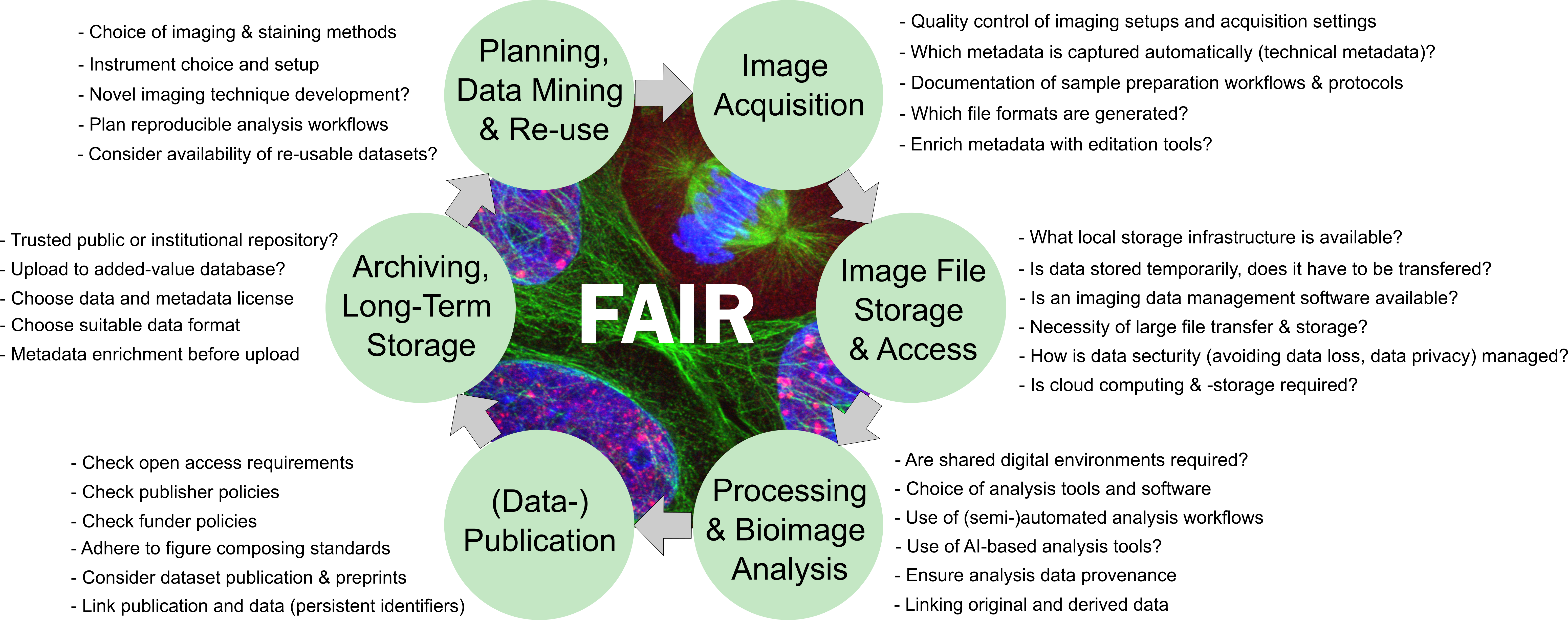
How to navigate the bioimage data life cycle?
Any experiment starts with proper planning. It is natural for researchers to think about experimental design in terms of specimen preparation, control groups vs. experimental groups, sample sizes, and the expected quantitative or qualitative research result to allow testing a given hypothesis. When the experiment involves bioimaging methods, the choice of contrasting methods (e.g., stainings, fluorescent marker dyes, light contrast methods, etc.), and the choice of a suitable imaging device are vital considerations. For example, if a cell line in a culture dish is imaged after fixation and staining, the choice of the microscope (e.g., confocal, widefield, superresolution microscopy) would naturally be different as compared to imaging whole (living) specimens in 3D (light sheet microscopy, intravital multi-photon microscopy). In addition, the image processing and analysis should not only be carefully planned with respect to sample sizes, but also for the suitability of the analysis method, the match between image analysis workflows and the microscopy method, and the way the data shall be represented to make the results easily understandable. All these aspects belong to the due diligence for good scientific practice. Using the data life cycle concept for planning the research data management ahead will greatly contribute at this stage. All of this planning should be collected in a data management plan before performing a study or an individual experiment.
Planning, data mining, and re-use
In this proposed data life cycle, planning and data mining for reusable data are combined as one life cycle step. While the optimal case for data mining would be finding a published dataset of images that allows making the desired analysis for the own research question on these images without the need for an own microscopy session… well, this scenario is probably unlikely for most researchers. So why bother with data mining? Before you spend hours of testing staining protocols, performing experiments with different microscopes and lenses, and, in the worst case, finding out after acquisition that your data is not fit for the analysis you aimed for, it can be helpful to search for comparable stainings, comparable analyses, and comparable microscope images in public repositories before you go the microscope. Use metadata to search for published data to find out how other researchers performed a similar experiment and the analysis before. You might even download other researchers’ raw images and run your intended analysis with them to make sure that your analysis will work once you have acquired your own images.
Before performing your own microscope session to acquire data, plan ahead along the steps of the life cycle. Make sure that not only you will be able to answer your research questions as intended but also that the process from data acquisition to the published results is fully tracked and understandable for your future self and for others (reproducibility and scientific rigor). Consider that maybe your dataset will be the valuable data mining resource for others in the future (findability, accessibility, interoperability, reusability, FAIR).
Data acquisition
Microscopes typically record technical metadata automatically and capture these in the image files (in metadata headers). However, researchers should carefully consider which metadata fields are vital to understand and reproduce the experiment and ensure that the images contain this information. Typical metadata include pixel size, objective lens, laser power, filter settings, etc. These are technical metadata relevant to the correct interpretation of the experiment. In addition, sample preparation and experimental metadata should be recorded. If these metadata are recorded in written lab notebooks, their digital representation must be ensured, too. For example, using an image data management platform like OMERO (or others) allows for the structured annotation of these important metadata. Electronic lab notebooks can be used to retrieve the experimental metadata in an open digital format. Also the image data files themselves should either be in an open format or be accessible without proprietary software via the use of conversion tools (e.g., Bio-Formats)
Data storage and access
An image is no end in itself. To review microscopy images, process the images, analyze them, and to share the images and analytical results with others, your data must be properly structured, and safely stored. When planning your experiment, think about and document how the data is transfered from the mircoscope computer to the storage place where you will be working with the data. How will the raw data be faithfully secured? How is data backed-up to avoid data loss? Who has access to the data and who owns the storage location? How do you handle derived images and analysis results and make sure these can be traced back to original raw data? Eventually, where will the data be stored long-term, who will have access even after the individual researcher has left the institution, and is there a date or event that will trigger data deletion? Engaging with your core facility and IT service providers to discuss these and related questions is recommended. An important aspect: Not only the storage location but also the network or cable access rates to this location for the purpose of using the data for analysis (hot data) should be taken into account.
Processing and analysis
Plan ahead for your image processing and analysis! Not only should you design the analysis workflow so that it will faithfully represent the biological event you intend to measure. It must also be ensured that the analysis steps are recorded properly and the path from raw to result can be reproduced. Have you ever read a paper’s methods section stating “image analysis was performed in Fiji” only? That is certainly not enough to let you redo the same analysis, right? For your experiment, make sure to consider how your data handling and metadata annotation influences the traceability through your analysis process, and how you document this process. More so, proper data handling can significantly ease your analysis workflow and help you streamline otherwise tedious tasks (see the comment by Tom Boissonnet here).
Data publication
When the time is ripe that your research is about to be published in a peer-reviewed journal – congratulations! This is what scientists strive for. In most classical (eletronic) paper publications you may find a statement saying “original data is available upon request” or even “all original data is included in this manuscript.” Looking more closely, you often end up realising that the presented “Fig 4.B.” image inlets are not really raw data that would allow reproducing the results shown in the bar chart next to it. Also, a systematic investigation found that most “data available upon request”, are, in fact, never available in real life (Gabelica, 2022). That said, it is recommended to plan ahead in which way your original data will be available for the public after acquisition. A good approach would be to think about an image data repository where you want to publish the data belonging to the publication. If your dataset contains even more valuable raw data than you have used yourself for the specific research question in any individual paper, you might want to consider publishing your data as a dataset publication.
Archiving, Long-term storage
Do you publish all the raw data belonging to your publication in a public repository? Then you might have killed this bird with that same stone already since a repository like, e.g., the BioImage Archive offers the long-term storage of the bitstream behind your images including the metadata records you have submitted. Commonly, however, research institutions offer long-term archiving, for example on tape storage systems where cold data is secured over many years. The downside of this storage alone is that nobody except the researchers have ususally access to restore the data back from the tape archive. Yet, this solution for long-term storage can fulfill the requirement to hold original raw data for at least ten years after publication of the results. Plan ahead and consult with your institutional IT deparment and (if available) with your data stewardship team to learn about how your data will ultimately be stored over the long-term.
Re-iterating the life cycle
Here we are, your data plan is fit for making your research data an excellent contribution to the scientific community as a whole. None of the above steps are trivial, and in some cases, your individual research needs might exceed what is technically available yet. But for sure, the effort of having your data management in mind throughout all the steps of the data life cycle will ultimately contribute to good science, and foremost, it will be an important courtesy for your future self when you realize that looking at that old dataset again will be of great value for your current research.